Cloud-Native Data Management Platforms
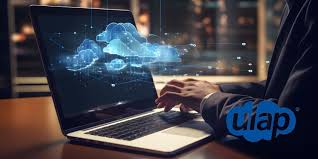
In recent years, businesses have witnessed a significant shift towards cloud-native data management platforms. This trend is reshaping the landscape of data management, offering organizations unprecedented scalability, flexibility, and cost-effectiveness. Cloud-native platforms leverage cloud computing infrastructure to provide on-demand resources and services, allowing businesses to scale their data operations dynamically based on changing needs. This approach eliminates the need for businesses to invest in and maintain on-premises hardware, reducing upfront costs and operational complexities. Moreover, cloud-native platforms enable seamless integration with other cloud services, facilitating a more holistic approach to data management.
Embracing Scalability and Flexibility
Cloud-native data management platforms offer unparalleled scalability and flexibility, allowing businesses to scale their data operations seamlessly as demand fluctuates. With cloud-native architectures, businesses can leverage auto-scaling capabilities to dynamically allocate resources based on workload requirements, ensuring optimal performance and cost-efficiency. This scalability is particularly beneficial for businesses with fluctuating data processing needs, such as those in e-commerce, finance, and healthcare. Additionally, cloud-native platforms provide flexibility in deployment options, supporting hybrid and multi-cloud architectures to accommodate diverse business needs and preferences.
Streamlining Data Operations
Cloud-native platforms streamline data operations by providing a unified and integrated environment for data storage, processing, and analytics. Businesses can leverage a wide range of cloud services and tools to build end-to-end data pipelines, from data ingestion to analysis and visualization. This integrated approach reduces complexity and eliminates the need for disparate tools and systems, enabling more efficient and streamlined data operations. Moreover, cloud-native platforms offer built-in support for popular data processing frameworks and languages, such as Apache Spark, Python, and R, empowering data engineers and analysts to work with familiar tools and languages.
Real-Time Data Processing and Analytics
In the digital age, businesses are increasingly reliant on real-time data insights to drive decision-making and gain a competitive edge. Real-time data processing and analytics capabilities are becoming a cornerstone of modern data management platforms, enabling businesses to analyze streaming data in real-time and derive actionable insights instantaneously. This trend is fueled by advancements in technologies such as in-memory computing, stream processing, and event-driven architectures, which enable businesses to process and analyze data at unprecedented speeds. Real-time data processing and analytics empower businesses to detect and respond to emerging trends, events, and anomalies in real-time, enabling more proactive and informed decision-making.
Harnessing the Power of AI and ML
Artificial intelligence (AI) and machine learning (ML) are revolutionizing how businesses manage and analyze their data, and data management platforms are no exception. Cloud-native platforms are increasingly integrating AI and ML capabilities to automate data processing tasks, uncover hidden patterns and insights, and enable predictive analytics. This trend is driven by the growing demand for AI-driven insights and predictions to inform strategic decision-making and drive business outcomes. By leveraging AI and ML algorithms, businesses can extract valuable insights from their data more efficiently and accurately, enabling them to make data-driven decisions with confidence and agility.
Data Privacy and Security
With the increasing volume and complexity of data being generated and processed, data privacy and security are paramount concerns for businesses. Cloud-native data management platforms prioritize data privacy and security by implementing robust encryption, access controls, and compliance measures to protect sensitive data from unauthorized access and breaches. Additionally, cloud-native platforms offer built-in support for data governance, data masking, and anonymization to ensure compliance with regulations such as GDPR and CCPA. By prioritizing data privacy and security, businesses can build trust with their customers and partners, safeguard their reputation, and mitigate the risk of regulatory fines and penalties.
The Evolution of Data Integration
ETL vs ELT…The debate between ETL (Extract, Transform, Load) and ELT (Extract, Load, Transform) approaches to data integration is evolving in the era of cloud-native data management platforms. Traditionally, ETL has been the preferred approach for batch processing and structured data, where data transformation occurs before loading into the target system. However, with the rise of cloud-native architectures and real-time data processing capabilities, ELT is gaining traction for its flexibility and scalability. In the ELT approach, data is loaded into the target system without significant transformation, allowing businesses to leverage the processing power of the target system for transformation and analysis. This approach is well-suited for modern analytics and big data scenarios, where raw data needs to be stored first, and transformation is performed later.
Navigating the Data Landscape
The future of business data is bright, with cloud-native data management platforms leading the way in driving innovation and transformation. By embracing cloud-native architectures, real-time data processing and analytics, AI and ML capabilities, and robust data privacy and security measures, businesses can unlock the full potential of their data and gain a competitive edge in the digital age. Moreover, the evolution of data integration approaches, from ETL to ELT, reflects the changing needs and preferences of businesses in managing and analyzing their data. As businesses continue to adopt cloud-native data management platforms and harness the power of their data, they will be better equipped to navigate the complexities of the modern data landscape and drive growth and innovation in their respective industries.